Publications
Teratology Primer, 3rd Edition
How Can Predictive Modeling Be Used to Assess Developmental Toxicity?
Nisha S. Sipes, National Toxicology Program, National Institute of Environmental Health Sciences, Research Triangle Park, North Carolina
What is Predictive Modeling?
A predictive model is a functioning method to forecast an outcome. In the case of developmental toxicity, the model would be used for toxicity prevention as we would want the model to predict what exposures would cause human developmental toxicity. The rodent model is the classic example of a predictive model for human developmental toxicity. It is an intact developing living system with the ability for the chemical to partition among tissues, to be broken down into fragments that may be less or more toxic than the parent compound (metabolism), to have multiple routes of exposures, and to reach biological targets that are directly related to mammalian, including human, development. There is a desire to reduce or eliminate the need for animal testing for ethical reasons, to decrease the time it takes to understand the exposure level at which a chemical is a potential toxicological concern, to reduce the cost associated with these studies, and to increase mechanistic understanding of how the chemical causes the toxicity.
As an example, pesticides in the United States require animal testing with specific guidelines and reporting protocols that need extensive oversight for regulation, leading to years before an exposure is reported as a potential concern for developmental toxicity with consequent increased cost. For pharmaceuticals, the results may be faster, but extensive testing still requires a significant number of animals and associated cost. Furthermore, it is known that some chemicals (e.g., thalidomide) do not elicit developmental toxicity effects similarly between human and rodent models. Faster and less expensive predictive developmental toxicity models are already in practice to mimic some aspects of developmental biology, including zebrafish embryos, and limb bud and whole embryo cultures, as discussed in another chapter of this Teratology Primer.
This chapter focuses on alternative high-throughput predictive models that integrate large amounts of information (e.g., high-throughput mammalian cell-based experiments with exposure to thousands of chemicals, data from public literature mining, and historical animal study data) on biological disruption due to specific chemical exposures, statistical methods, and mathematical modeling of the likely fate of chemicals in a simulated human. While these approaches apply to a variety of compounds, environmental chemicals are the focus, because thousands of non-pharmaceutical chemicals are in commerce today with little or no toxicological information. We can prioritize the most likely developmentally toxic exposures for further evaluation with the help of predictive models.
Data for High-Throughput Predictive Modeling
For high-throughput developmental toxicity prediction, a relational database is needed to find and sort connections between the input and output. Text-mining peer-reviewed literature data, through public sources such as PubMed, can pull relevant information about chemical-biological interactions from animal, cell-based, or cell-free studies. The Comparative Toxicogenomics Database has collected this information in a curated fashion to make connections between chemicals and genes, genes and diseases, and chemicals and diseases, noting the literature reference for cross-checking. Information specifically on developmental toxicity identified from animal studies can be found in publicly available governmental databases, such as the US EPA’s Toxicity Reference database (ToxRefDB), which is mostly from pesticide registration studies, and the National Toxicology Program’s (NTP) Chemical Effects in Biological Systems (CEBS) database. High-throughput screening (HTS) assays are being used to identify the potential for chemicals to alter biological processes as part of the Federal Tox21 partnership among the four federal agencies (US EPA, NTP, National Center for Advancing Translational Sciences, US Food and Drug Administration) and the US EPA’s ToxCast program. Specifically, these programs have screened over 8000 chemicals in >60 HTS assays and >1000 chemicals in >800 assays, respectively. These chemicals include those that have been historically tested in developmental toxicity animal tests. The assays range in complexity from cell-free receptor binding or enzyme inhibition, cell-based disruption of intracellular signaling, co-cultures of multiple cell types to mimic a more tissue-like environment simulating disruption of feedback among the cells, to zebrafish embryo cultures.
Predictive Models Using Biology and Statistics
Can we use a set of HTS assays to predict if an exposure will be developmentally toxic? If we can, then in the future, in lieu of the animal studies, we may be able to test chemicals in the subset of HTS assays that are predictive of developmental toxicity. In order to answer the question, we first need data on a set of chemicals that have been run in both HTS assays and chemical-animal studies. We next try to find patterns in the data, and ask questions such as, “if a group of chemicals lead to a particular developmentally toxic effect (e.g., cleft palate), do they affect the same HTS assays?” This question is addressed through statistical correlations. We can then use these assays to predict the developmental toxicity outcome, but we may also be able to better understand the biology on how those chemicals lead to the developmental toxicity outcome. We have performed this analysis on the Tox21/ToxCast data and ToxRefDB animal studies and found associations between assays and endpoints (such as transforming growth factor beta and cleft palate), differences between species (e.g., exposures affecting rabbits tended to be more associated with altering inflammatory pathways in the HTS assays), and associations among groups of endpoints (e.g., urogenital and palate defects are correlated). Another way to use these assays for predictive biology is to map the assays to pathways affecting developmental toxicity, often identified by expert judgement. Exposures that affect a majority of these pathways can be flagged and prioritized for evaluation of developmental toxicity. Using these datasets, this evaluation has been performed for vasculogenesis. While these approaches provide a way to prioritize exposures as developmentally toxic, other processes, such as the growth factor gradients important in development, are not captured by these HTS assays and may warrant further studies. Along these lines, another chapter in the Teratology Primer focuses on computer simulations of developmental processes using HTS data to figure out where in the process a chemical may cause an effect and how that effect disrupts a particular developmental process.
Predictive Models Using Biology and Kinetics
Kinetic models can build upon the statistical predictive models by incorporating data on how much of the chemical gets absorbed into the animal, how it gets distributed in the plasma and throughout the body, how it gets broken down through metabolism, and how the chemical exits the body or gets accumulated (collectively known as ADME). Unlike the situation in HTS assays, in the body, chemicals may be broken down or exit quickly, accumulated, or metabolized into more toxic metabolites. High-throughput toxicokinetic (HTTK) models allow the rapid processing of thousands of chemicals with a few chemical specific parameters, e.g., LogP, molecular weight, metabolic clearance (estimated or measured), fraction of the chemical unbound in plasma (estimated or measured). These models can take a HTS chemical-assay pair output, such as the concentration it takes to reach a 50% activation or inhibition of a target (AC50), and convert it to an estimated daily dose. The models can also take a dosing scenario (e.g., the subject is exposed to a dose of a specific compound 3 times a day for 5 days) and estimate internal plasma or specific tissue concentrations. These models allow us to take the internal body kinetics into consideration. An adult HTTK model is available, while a HTTK maternal-fetal specific model is under development. In addition, lower throughput chemical-specific kinetic maternal-fetal models containing more chemical-specific information have been and can be developed with the proper input information. Once the HTS chemical-assay pair concentration information is turned into an estimated dose, these doses can be directly compared to actual exposure information, if available. If the actual exposure is higher than what is estimated from the HTS data, the person or animal is exposed to doses for which the chemical may elicit a biological response, as indicated by the HTS assay.
Future of Predictive Modeling
While these two high-throughput predictive modeling efforts can rapidly prioritize thousands of compounds and decrease cost and animal use, there are more questions to be asked. Aspects not covered in these models include 1) is the biological space covered adequately in the HTS assays? 2) what is a “developmental toxicant” (i.e., dose should be taken into consideration)?, 3) how does the changing metabolism and interaction with the fetus affect developmental toxicity?, and 4) how do the placenta, blood-brain barriers, and transporters affect the chemical distribution? While there is always more research to do, these models have proven useful. For example, the US EPA has adopted a predictive model using a series of estrogen-related HTS assays, in lieu of an animal model, toward regulating chemicals interacting with the body’s endocrine system. There is a future for high-throughput predictive modeling, and it is ripe for research. There is much to learn and much to discover.
The National Toxicology Program Division within the National Institute of Environmental Health Sciences funded and managed the research described and this paper has been subjected to review and approved for publication. Reference to commercial products or services does not constitute endorsement.
Suggested Reading
National Academies (2007). Toxicity testing in the 21st century: a vision and a statement. United States, The National Academies Press. 216 pp.
Silver N (2012). The Signal and the Noise: Why So Many Predictions Fail – but Some Don't. United States, Penguin Press. 534 pp.
Knudsen T, Martin M, Chandler K, Kleinstreuer N, Judson R and Sipes N (2013). Predictive Models and Computational Toxicology. In: Teratogenicity Testing: Methods in Molecular Biology. Edited: P Barrow, Humana Press, New York., 947:343-74.
Sipes NS, Wambaugh JF, Pearce R, Auerbach SS, Wetmore BA, Hsieh JH, Shapiro AJ, Svoboda D, DeVito MJ, Ferguson SS. (2017). An Intuitive Approach for Predicting Potential Human Health Risk with the Tox21 10k Library. Environ Sci Technol 51(18), 10786-10796.
Figures
Figure 1. Building a predictive model for developmental toxicity from in vitro high-throughput screening data to predict an in vivo animal outcome or developmental process requires finding patterns in a database containing chemical-specific data between both outcomes.
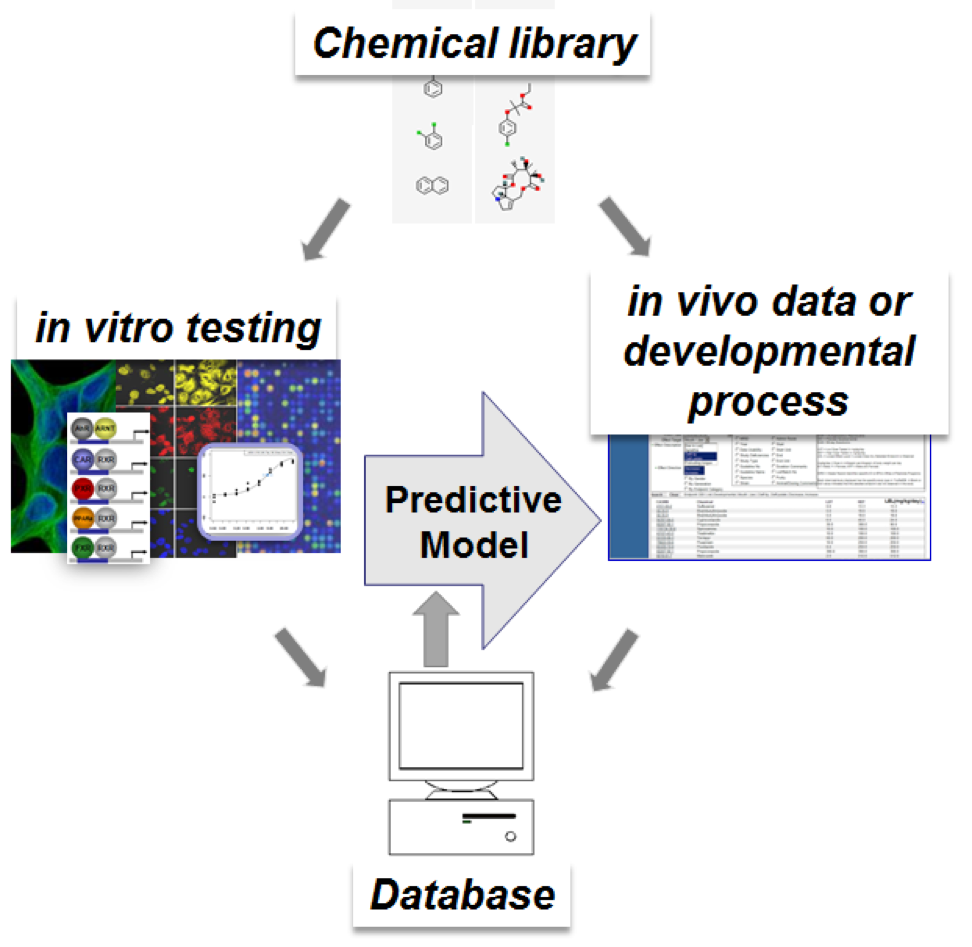
Figure 2. High-throughput toxicokinetics can rapidly convert chemical exposure doses to internal concentrations as well as high-throughput screening concentrations (e.g., AC50) into doses, while high throughput screening data can provide mechanistic information about a chemical’s interaction with biology.
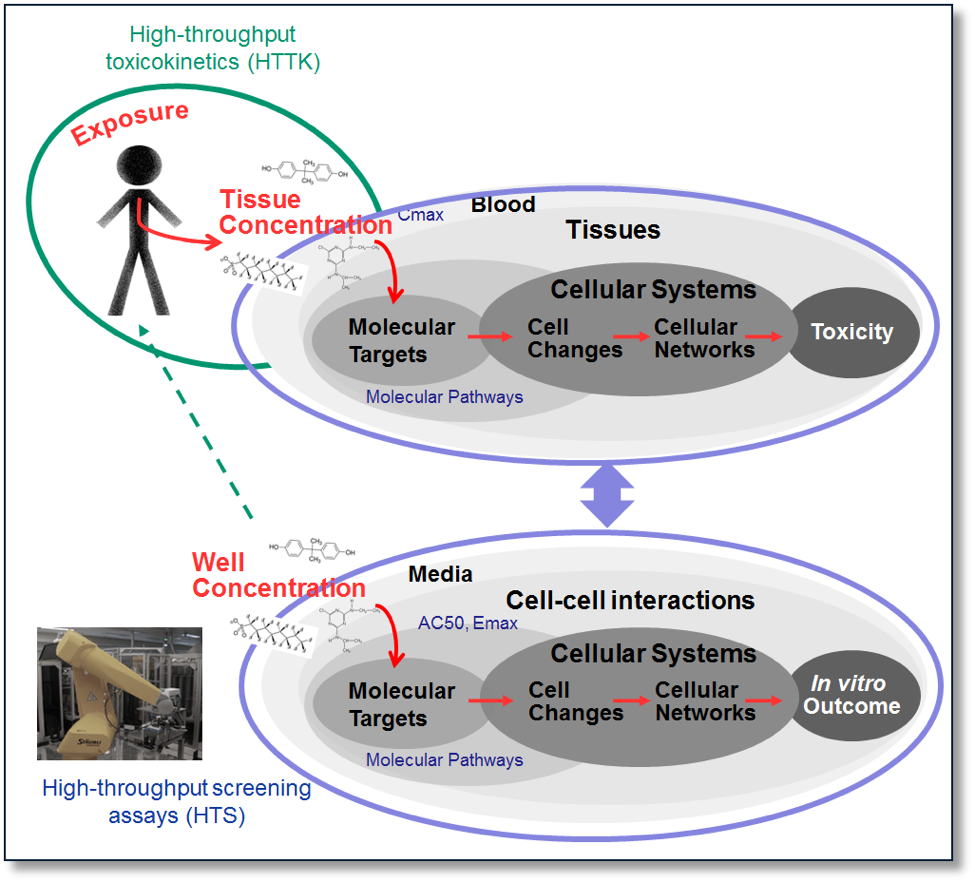